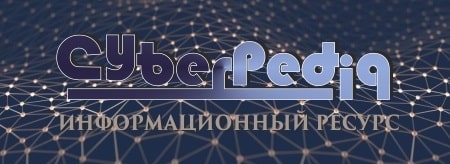
Особенности сооружения опор в сложных условиях: Сооружение ВЛ в районах с суровыми климатическими и тяжелыми геологическими условиями...
История развития пистолетов-пулеметов: Предпосылкой для возникновения пистолетов-пулеметов послужила давняя тенденция тяготения винтовок...
Топ:
Организация стока поверхностных вод: Наибольшее количество влаги на земном шаре испаряется с поверхности морей и океанов...
Установка замедленного коксования: Чем выше температура и ниже давление, тем место разрыва углеродной цепи всё больше смещается к её концу и значительно возрастает...
Когда производится ограждение поезда, остановившегося на перегоне: Во всех случаях немедленно должно быть ограждено место препятствия для движения поездов на смежном пути двухпутного...
Интересное:
Влияние предпринимательской среды на эффективное функционирование предприятия: Предпринимательская среда – это совокупность внешних и внутренних факторов, оказывающих влияние на функционирование фирмы...
Что нужно делать при лейкемии: Прежде всего, необходимо выяснить, не страдаете ли вы каким-либо душевным недугом...
Отражение на счетах бухгалтерского учета процесса приобретения: Процесс заготовления представляет систему экономических событий, включающих приобретение организацией у поставщиков сырья...
Дисциплины:
![]() |
![]() |
5.00
из
|
Заказать работу |
|
|
The history of MT began in 1666, when Leibniz in his dissertation, The Art of Combinations, had clearly indicated the possible mechanization of both arithmetic and thought processes, that his logical processor could be used to transform one language into another. He also considered the extraction of ideas from text and their expression in terms of a metalanguage (Saw 1954). The culmination occurred in his design of the first practicable mechanical multiplying machine (1694) and in a binary multiplier (Eriksson et al. 1996). On June 20, 1946, New York the discussion between Weaver and A.D. Booth identified the fact that the code-breaking process in no way resembled language translation because it was known a priori that the decrypting process must result in a unique output. The main purpose of this meeting, however, was to interest the Rockefeller Foundation in supporting development of an electronic computer at the University of London. In March 1947, Warren Weaver, director of the Division of Natural Sciences of the Rockefeller Foundation, in correspondence with Andrew D. Booth and Norbert Wiener first formulated the concept of machine translation.
The earliest “translation engines” were based on a direct, so called “Transformer” approach. Input sentences of the source language were transformed directly into output sentences of the target language. At first the machine did a rough analysis of the source sentence dividing it into subject-object-verb, etc. Then source words were replaced by target words selected from a dictionary and their order was rearranged according to the rules of the target language. These rough operations were resulted in a simplified transformation with lots of silly sentences so much laughed at now. To process any translation, human or automated, the meaning of a text in the original (source) language must be fully restored in the target language, i.e. the translation. While on the surface this seems straightforward, it is far more complex. Translation is not a mere word-for-word substitution. A translator must interpret and analyze all of the elements in the text and know how each word may influence another. This requires extensive expertise in grammar, syntax (sentence structure), semantics (meanings), etc., in the source and target languages, as well as familiarity with each local region. Human and machine translation each have their share of challenges. For example, no two individual translators can produce identical translations of the same text in the same language pair, and it may take several rounds of revisions to meet customer satisfaction. But the greater challenge lies in how machine translation can produce publishable quality translations.
The ideal aim of machine translation systems is to produce the best possible translation without human assistance. Basically every machine translation system requires programs for translation and automated dictionaries and grammars to support translation. Machine translation systems that produce translations between only two particular languages are called bilingual systems and those that produce translations for any given pair of languages are called multilingual systems. Multilingual systems may be either uni-directional or bi-directional. Multilingual systems are preferred to be bi-directional and bi-lingual as they have ability to translate from any given language to any other given language and vice versa.
|
If talking about rule-based machine translation, it relies on countless built-in linguistic rules and millions of bilingual dictionaries for each language pair. The software parses text and creates a transitional representation from which the text in the target language is generated. This process requires extensive lexicons with morphological, syntactic, and semantic information, and large sets of rules. The software uses these complex rule sets and then transfers the grammatical structure of the source language into the target language. Translations are built on gigantic dictionaries and sophisticated linguistic rules. Users can improve the out-of-the-box translation quality by adding their terminology into the translation process. They create user-defined dictionaries which override the system's default settings. In most cases, there are two steps: an initial investment that significantly increases the quality at a limited cost, and an ongoing investment to increase quality incrementally. While rule-based MT brings companies to the quality threshold and beyond, the quality improvement process may be long and expensive.
Statistical machine translation utilizes statistical translation models whose parameters stem from the analysis of monolingual and bilingual corpora. Building statistical translation models is a quick process, but the technology relies heavily on existing multilingual corpora. A minimum of 2 million words for a specific domain and even more for general language are required. Theoretically it is possible to reach the quality threshold but most companies do not have such large amounts of existing multilingual corpora to build the necessary translation models. Additionally, statistical machine translation is CPU intensive and requires an extensive hardware configuration to run translation models for average performance levels. Rule-based MT provides good out-of-domain quality and is by nature predictable. Dictionary-based customization guarantees improved quality and compliance with corporate terminology. But translation results may lack the fluency readers expect. In terms of investment, the customization cycle needed to reach the quality threshold can be long and costly. The performance is high even on standard hardware. Statistical MT provides good quality when large and qualified corpora are available. The translation is fluent, meaning it reads well and therefore meets user expectations. However, the translation is neither predictable nor consistent. Training from good corpora is automated and cheaper. But training on general language corpora, meaning text other than the specified domain, is poor. Furthermore, statistical MT requires significant hardware to build and manage large translation models.
Rule-Based MT | Statistical MT |
+ Consistent and predictable quality | - Unpredictable translation quality |
+ Out-of-domain translation quality | - Poor out-of-domain quality |
+ Knows grammatical rules | - Does not know grammar |
+ High performance and robustness | - High CPU and disk space requirements |
+ Consistency between versions | - Inconsistency between versions |
- Lack of fluency | + Good fluency |
- Hard to handle exceptions to rules | + Good for catching exceptions to rules |
- High development and customization costs | + Rapid and cost-effective development costs provided the required corpus exists |
Given the overall requirements, there is a clear need for a third approach through which users would reach better translation quality and high performance (similar to rule-based MT), with less investment (similar to statistical MT). http://www.systran.co.uk/
|
6. Find and learn Russian equivalents for the following words and expressions:
1) rule-based | a) | ||
2) user-defined | b) | ||
3) statistical machine translation | c) | ||
4) bilingual corpora | d) | ||
5) source language | e) | ||
6) target language | f) | ||
7) quality threshold | g) | ||
8) binary multiplier | h) | ||
9) decrypting process | i) |
7. Find and learn English equivalents for the following words and expressions:
1) необычный, исключительный | a) | k) | |
2) установки (параметров) по умолчанию | b) | l) | |
3) устойчивость системы | c) | m) | |
4) качество внешней среды (в которой должна работать программа) | d) | n) | |
5) обеспечение словаря, основанное на конкретных требованиях отдельного клиента | e) | o) | |
6) встроенные грамматические правила | f) | p) | |
7) дословная замена (подстановка) | g) | q) | |
8) процесс дешифрации сообщений | h) | r) |
Metalanguage
A form of language or set of terms used for the description or analysis of another language.
|
|
Типы оградительных сооружений в морском порту: По расположению оградительных сооружений в плане различают волноломы, обе оконечности...
Двойное оплодотворение у цветковых растений: Оплодотворение - это процесс слияния мужской и женской половых клеток с образованием зиготы...
История развития хранилищ для нефти: Первые склады нефти появились в XVII веке. Они представляли собой землянные ямы-амбара глубиной 4…5 м...
История развития пистолетов-пулеметов: Предпосылкой для возникновения пистолетов-пулеметов послужила давняя тенденция тяготения винтовок...
© cyberpedia.su 2017-2024 - Не является автором материалов. Исключительное право сохранено за автором текста.
Если вы не хотите, чтобы данный материал был у нас на сайте, перейдите по ссылке: Нарушение авторских прав. Мы поможем в написании вашей работы!